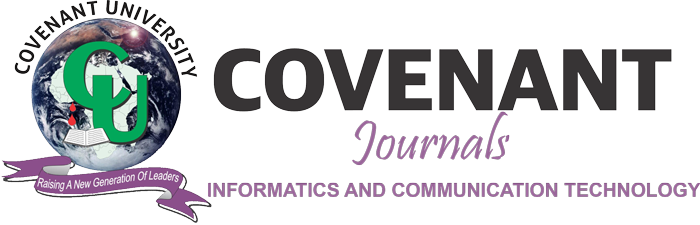
Concerns have been expressed over Internet of Things (IoT) devices' growing prevalence and susceptibility to cyberattacks, namely Man-in-the-Middle (MitM) assaults. The performance of selected machine learning algorithms: Logistic Regression, Decision Trees, and K-Nearest Neighbors were analyzed and compared using accuracy, precision, recall, F1-score, and error rate using a dataset comprising normal and attacked data sets from Kaggle. According to the research findings, the Decision Tree algorithm outperformed other selected algorithms in terms of MitM attack prediction accuracy of 99.42% and a good balance between precision, F1-score, and recall, with the lowest error rate of 0. 0058. The results of the study improve the security and reliability of IoT applications by aiding in the creation of efficient MitM attack prediction systems for IoT environments. The findings also emphasize how crucial it is to choose the best machine-learning algorithm for a given IoT security task. Investigating the use of transfer other techniques in MitM attack detection for IoT contexts is one area of future research.