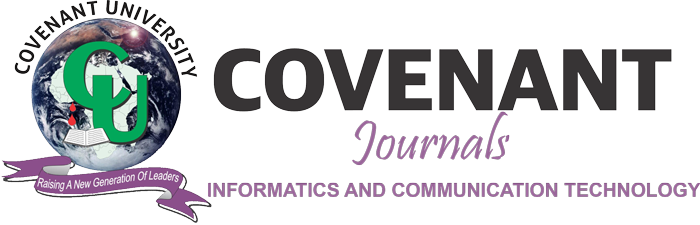
Diabetic Retinopathy (DR), a leading cause of visual impairment among working-age adults, is increasing globally, necessitating effective predictive tools. Machine learning (ML) classifiers often struggle with high-dimensional datasets, making feature selection (FS) critical for improving predictive performance. This study evaluates the impact of FS techniques on the performance of an ML model for DR prediction using the MESSIDOR retinal dataset. Two FS methods, forward selection and variance threshold, were compared alongside a multilayer perceptron (MLP) classifier. The results showed that forward selection significantly enhanced MLP accuracy to 77.06%, outperforming the raw dataset (75.32%) and variance threshold (73.16%). The findings underscore the importance of appropriate FS in developing robust ML models. Integrating such models into clinical workflows could enhance early DR diagnosis, facilitate timely treatment, and reduce the risk of severe visual impairment, ultimately improving patient outcomes and healthcare efficiency.