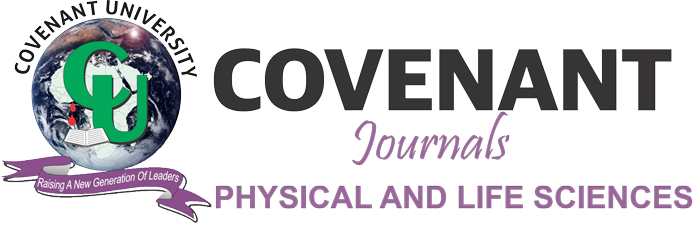
Innovation distributions play significant role in determining the fitness as well as forecasting performance of volatility models. Several studies aimed at comparing the performance of volatility have been carried out but most of the studies focused on the use of Gaussian innovation distribution. Hence, this study compares the performance of GARCH models and its extensions using five innovation distributions, one Gaussian distribution (normal distribution) and four non- Gaussian innovation distributions(Student –t distribution, generalized error distribution, skewed Student- t and skewed generalized error distribution). Data on the daily closing prices of Zenith bank (04/01/2007 to 31/12/2019) and ETI (04/01/2007 to 31/12/2019) were obtained from cashcraft website and then converted to daily returns. Hence, using these five innovation distributions, the parameters of GARCH(1,1), TGARCH(1,1), EGARCH(1,1), IGARCH(1,1) and GJR- GARCH(1,1) were estimated. The performances of these models were compared in terms of fitness using AIC and forecasting performance based on Root Mean Square Error. Result of analysis revealed that GARCH models and its extensions estimated using non- Gaussian innovation distributions outperformed other innovation distributions both in terms of fitness and forecasting accuracy. Result also shows that among the non-Gaussian innovation distributions considered, the skewed generalized error distribution performed better than other non-Gaussian innovation distributions. The TGARCH (1,1)-sged and E-GARCH (1,1)-sged were recommended as the best model for predicting the volatility in ETI and Zenith bank stocks respectively.