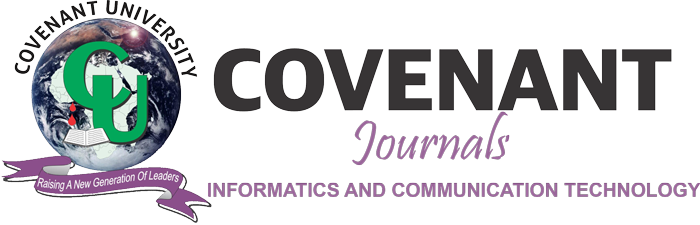
Introduction: Optimisation is an important aspect of machine learning because it helps improve accuracy and reduce errors in the model's predictions.
Purpose: The purpose of this research is to identify the global structure of optimization and machine learning. The work specifically looks at the collaborative network of countries in these fields, the top 20 authors in terms of production from 2015–2021, and the co-citation network of articles.
Methodology: In this study, co-word analysis and social network analysis were used to conduct a descriptive study based on the scientometric approach and the content analysis method. In this research, around 17,500 articles on optimization and machine learning published between 2015 and 2021 were extracted. An ANOVA was performed to evaluate whether there was a significant difference between betweenness, closeness, and pagerank. The Dimensions database was utilised for the investigation without language constraints. Moreover, Bibliometrix was used for calculation and visualization.
Findings: The results revealed a substantial difference between betweenness, proximity, and pagerank, indicating that this research has the potential to bring vital insights into future optimization and machine learning research.