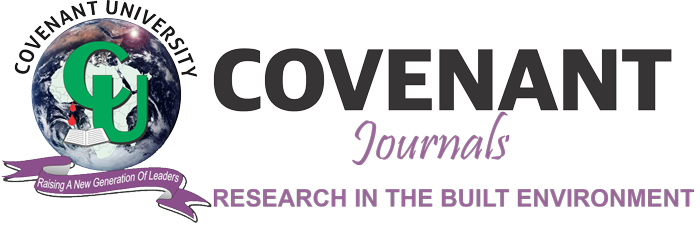
Building cost informatics is a body of knowledge that involves application of computer, digital system, building information modeling and state of art software in solving cost issues in building works and presentations in this study adopted building cost-informatics system in developing a model. The aim of the study is to generate a cost prediction model for residential building using a combination of parametric regression method and neural network (an expert system).The main objective of the work is to generate a stable cost prediction algorithm and model from neural network and regression method. Neural network is a conventional method currently being used in cost modeling, given its advantage over traditional regression method the objective is to use the strength of the two methods to generate an hybrid model that could be used in holistic cost prediction of residential building works. To achieve this, One hundred (100) samples of residential building projects were selected at random and divided into two; one part is used in developing network algorithm while the second part is used for model validation. Neural network is used to generate an optimized cost prediction algorithm which was divided into modules: the data optimization module, criteria selection with initializing and terminating modules. The generated algorithm and model was validated with Regression analysis was carried out and Jackknife re-sampling technique. It was discovered from colinearity analysis that there was high level of tolerance and -0.0756 lowest variation prediction quotients to 0.8678 highest variation quotients. Also the Regression coefficient (R-square) value for determining the model fitness is 0.069 with
standard error of 0.045. These results attests to the fitness of the model generated. Implication of the above data is that the model algorithm is a stable one which could be processed further into software, it is flexible and adaptive in accommodating new data and variables, thus, it allows for continuous updating.