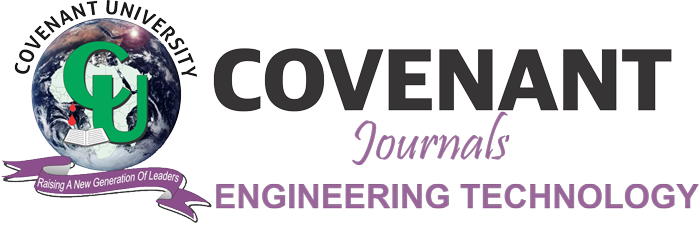
This paper explores an intelligent classification of different materials from their sound properties irrespective of shape, texture or size. This is towards the building of smart devices particularly useful in waste sorting and recycling. The selected materials are of three broad categories namely metals, glass and plastic. Pre-processing involves filtering noise from the captured sound data, application of principal component analysis (PCA) was carried on extracted frequency and bandwidth feature vectors with the aim of extracting the characteristic properties that contribute the most to variance in order to improve classification accuracy of the training samples. Some common classifiers were tested with the data for accuracy of classification. These include KNN, Random Forest, Adaboost, SVM, Neural Network. KNN gave the best classification accuracy of 96.8%, while the Support Vector Machine (SVM) gave the least performance. By including the band width data for the three materials, it was observed that better identification of materials was achieved.Â